AI and ML are two rising technologies being used by several companies today. Have a look at their benefits for networking!
AI and ML for Network Automation
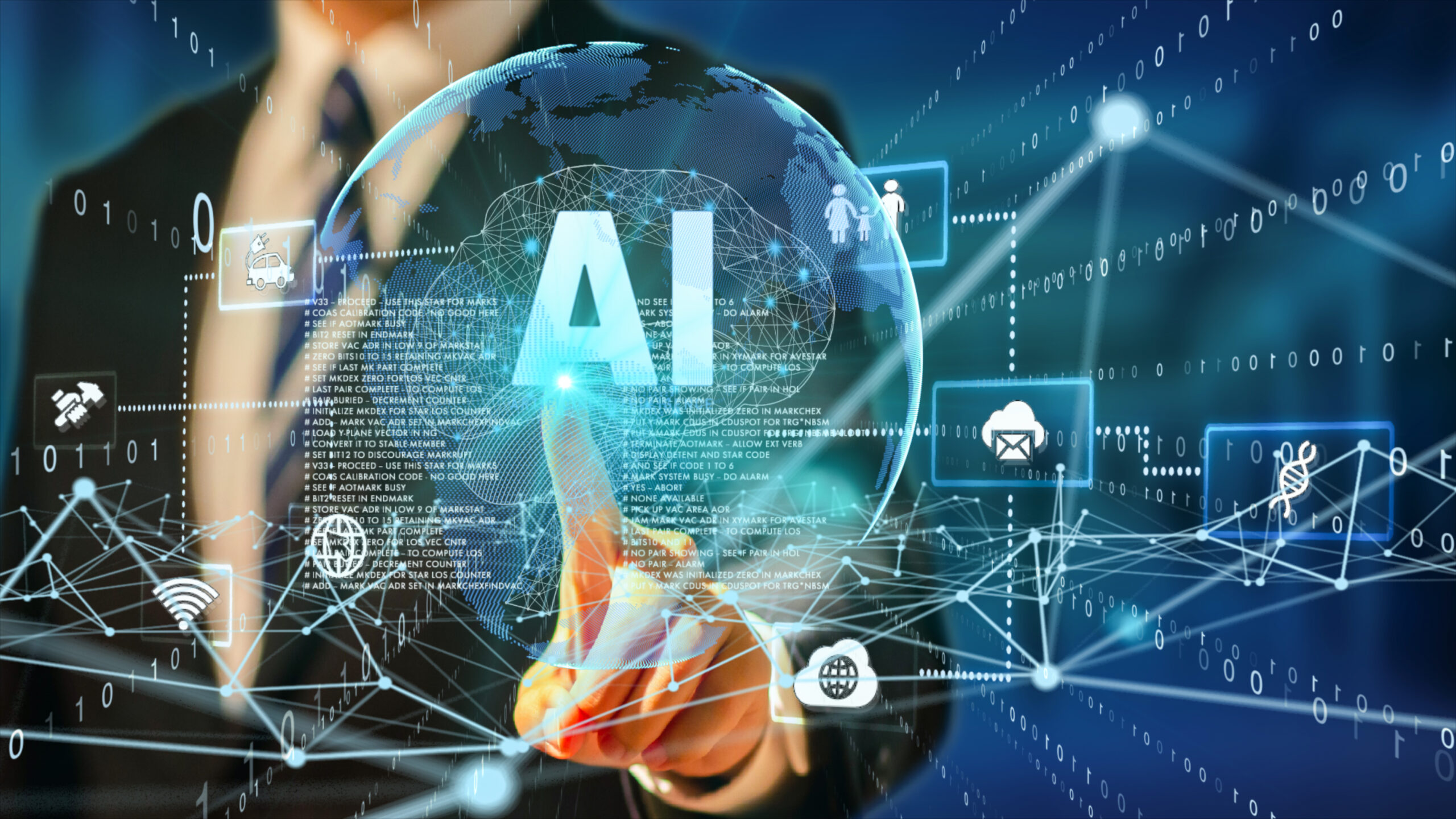
AI and Network Automation
The incorporation of artificial intelligence (AI) and machine learning (ML) in network design continues to expand. It is anticipated that most businesses will adopt some degree of automation within the next few years.
While many businesses are only beginning their journeys toward automation, others have already seen notable success with their initial deployments. AI and ML help with better decision-making and process automation when used in complicated IT operations. Humans’ decision-making and reaction times are often insufficient for handling an enterprise network because we cannot foresee or regulate every scenario.
ML and AI can conquer these drawbacks by providing insights based on historical data that can be implemented to current circumstances with unparalleled speed and precision. Let’s examine the working and benefits of these two technologies for network automation.
Automated Networking: An Introduction
Automating the preparation, deployment, processes, and optimization of networks and their systems constitutes network automation. At a fundamental level, network automation solutions automate the manual procedures and tasks conducted at each phase of the network lifecycle by transferring them to software applications that can accomplish them reliably and repeatedly.
The objective is for networks to modify and enhance themselves autonomously in response to real-time traffic patterns, configurations, software updates, and other factors. When it comes to Autonomous Networking, it’s all about the never-ending cycle of monitoring, analyzing, and optimizing processes with minimal human involvement. Automating networks via software-defined networking (SDN) falls under this category.
Congestion prevention, dynamic control, increased traffic forwarding, rapid system failures, and other real-time network events can all be mitigated with the help of big data and insights in real-time. Machine learning applied to a highly complex data set could be used to proactively reroute traffic, saving money for business owners by reducing the need for wasteful infrastructure upgrades.
Operators are embracing the virtualized future by launching cloud platforms with SDN/NFV. Cloud operations face a significant challenge in managing the entire life cycle of these virtual networking functions (VNFs) across hybrid cloud platforms.
In an outage, AI and ML-based systems can automatically scale to meet demand and repair any damage they may have sustained. These methods can also decide where VNFs should be deployed regarding the company’s compliance and safety standards.
When running a network, bugs will always be present. Network centers of the future will use techniques from artificial intelligence to better understand how current events compare to past ones. Then, they can utilize pattern-matching methods to intelligently group alarms from different domains to spot incidents, guide what to do next, and launch corrective measures. In the future, networks will be managed almost entirely by themselves, with impending fault conditions estimated and disciplinary actions taken automatically.
What can Automated Networking Achieve for your Business?
Businesses want to integrate their IT systems and applications as efficiently as possible. Additionally, it has been challenging to integrate all of these components into a single application because each firm employs a separate technology from a different supplier.
Today, companies are pursuing growing usage of automation software such as orchestration systems and policy-based solutions that execute across several devices simultaneously. All of this comes as a result of the demand for easy integration.
Network automation should enable you to reduce errors, boost productivity, and expedite difficult operations if implemented properly. Automated networks can significantly increase operational effectiveness for most enterprises thanks to their cheaper costs, better speeds, and increased agility. In addition, automation also allows staff to concentrate on higher-level concerns, like evaluating possible problems and resolving network-wide difficulties.
With AI and ML, businesses can react to changes fast. If your software for network monitoring is automated, you will be able to spot problems within seconds rather than days or weeks. Additionally, automation allows businesses to monitor hundreds of devices concurrently. Finally, an automated system ought to experience fewer failures than one that depends on human intervention.
Artificial Intelligence in Networking
Automation is the use of machinery to conduct operations in accordance with pre-programmed criteria, typically for everyday activities. AI, which is commonly thought to be the emulation of the higher-order functions of humans in domains like visual processing, language understanding, and analytics, is the capacity of a machine or a computer to replicate human labor through training and automation.
AI systems can make judgments as a result of the application of sophisticated analytical approaches When used in conjunction with automatically advanced feedback loops. However, AI must exempt software systems that use traditional determining algorithms, such as those in which a specific process is constructed by one or more humans and is not dependent on AI techniques or tools. This is because AI should incorporate a substantial degree of learning from data, either as the entire process or as a discernible portion of the process.
Systems with artificial intelligence can carry out tasks automatically. AI systems adapt over time based on information and rules and constantly learn and develop. For instance, some AI algorithms will perceive real-world activity through simulation before implementing what it understands to situations that are similar in the future.
In essence, software that accomplishes a task just like a human expert is the simplest definition of artificial intelligence. AI is becoming increasingly important in managing the complexity of expanding IT networks.
It is now more difficult than ever to handle IT infrastructures because of the emergence of devices, information, and users. Businesses need to have a way to deal with this complexity because most IT finances are flat or declining, and many are now turning to artificial intelligence for assistance.
AI’s development is fueling a wave of technologies that influence how individuals connect with organizations, interact, and operate. The pace of AI is growing in the mobile industry. It is not surprising that many top organizations in the larger digital environment have already accepted the technology and announced their AI guidelines, given the technology’s broad effect and revolutionary nature.
Establishing an AI System
IT cannot cope with today’s demanding network requirements without the proper AI strategy. Here are a few technological components that an AI strategy must have.
Data
Massive quantities of high-quality data are the foundation of any functional AI solution. AI gathers and analyzes data over time to progressively increase its intelligence. Real-time data collection from all edge devices is essential for practical uses involving highly dispersed edge devices, like mobile devices and IoT. The data must then be processed quickly using AI algorithms.
Competence in a Particular Domain
These metadata chunks assist AI in decomposing the problem into manageable chunks that can be taught to the AI models. Design intent metrics and organized data classifications for classifying and tracking the wireless customer experience can be used to accomplish this task.
Toolbox for Data Science
The problem can be broken up into chunks of metadata specific to each domain. These chunks of metadata are prepared to be served into the potent universe of big data and ML. Various methods, such as neural networks and unsupervised or supervised ML, should be used to analyze the data and offer helpful insight.
Virtual Assistant for Networks
An ML technique called collaborative filtering is used to provide suggestions for related movies on Netflix or products on Amazon. In addition to making suggestions, collaborative filtering can be used to examine vast data sets to find and link the elements that make up an AI solution to a specific issue.
The virtual network assistant in AI could work in a wireless setting as a virtual wireless expert who helps solve challenging issues. Consider a virtual network assistant that uses high-quality data, subject-matter knowledge, and syntax to provide predictive tips on avoiding issues. It can pick up on subtleties in wireless networks and answer queries like “What went down?” and “Why did this occur?” These automated technological advancements are made possible by AI in networking.
What impact does AI have on 5G Network Automation?
Automation of 5G networks should reduce the possibility of mistakes associated with provisioning and launching networks at scale once most of the network slicing, coordination, and management tasks are moved on to AI systems. Businesses would enhance efficiencies without excessive disruptions or downtime. As network operators can proactively deal with fires at lower prices, customer service statistics should strengthen.
AI and networking also allow users to spot consumption and performance patterns in networks that they might not have previously noticed. Long-term trend analysis enables businesses to identify cutting-edge efficiencies. As the main driver for many technologies that rely on apps with high bandwidths and low latencies, the commitment of 5G is reviving the game plan.
Machine Learning in Networking
Machine learning, a branch of artificial intelligence, enables computers to understand without explicit programming. ML uses massive databases and algorithms to teach computer systems how to make decisions. Its algorithm gets smarter and more efficient as the number of datasets increases.
Machine learning, which uses algorithms to analyze data, gain knowledge from it, and arrive at a conclusion or prediction without specific guidelines, is necessary for AI to succeed. Deep learning, which uses neural networks for further understanding and automation, is a more complex and detailed model of ML that has recently emerged due to improvements in storage and computation abilities.
Machine learning performs particular tasks using statistical techniques, frequently requiring less data. As a result, machine learning can be carried out by systems that are on the lower end. ML Applications take longer to confirm the authenticity of results, but training them is quicker.
On the other hand, deep learning uses artificial neural networks, which need a larger volume of data to build models. In turn, this necessitates powerful GPUs. Deep learning frequently takes longer to train than to test due to its reliance on big data sets.
Benefits of ML in Networking
Managing network performance
ML tools can help with capacity planning and managing traffic. The tools can set up automatic or manual direct management replies to rectify the mistake after identifying when traffic peaks in some trails or keeps failing in others.
For instance, ML-driven route analytics may redirect traffic from links using an ISP undergoing a brownout to connections using other providers. Depending on the traffic circumstances, tools with ML could move half of the traffic going to a back-end framework from one computer system to another.
Network Analytics
ML tools can forecast traffic patterns in ways that help in decision-making in the future. Occurrences where it might be advantageous to use an ML tool to analyze traffic flows should be assessed by network professionals, for instance, in the following cases:
- Is the data center’s traffic switching from rack-to-rack towards server to server?
- Is there a change in traffic from many small-packet flows to fewer large-packet flows?
By spotting trends, IT can decide what varieties of networks to construct, like switch-based mesh, leaf-spine, or host-based mesh. The more data ML tools can access from all networks, the more thorough their assessment and suggestions can be. Root cause analysis benefits significantly from the use of ML tools.
The skills to connect with the systems more quickly and easily can be achieved by incorporating ML-driven data analysis with other AI tools, such as natural language processing. Virtual assistants can be built to assist system administrators in identifying and resolving problems with network problems.
Safety in a Network
ML is beneficial in the area of network safety. Any element in a network can be subjected to a behavioral analysis using ML tools. ML techniques have significantly improved behavioral analytics by drastically reducing the number of untrue positive reports. Attacks that span multiple layers of enterprise activity, like phishing emails, account compromise, Layer 7 threats on web apps, and OS-level connectivity compromise, become easier to detect.
Finding a vulnerable hardware node or even a malicious worker on the company network can be aided by network security teams by observing discrepancies in network behavior. Tools powered by machine learning can also be used to identify and mitigate distributed denial of service attacks.
Health Management
Data analytics powered by machine learning can monitor a network’s health and identify nodes showing signs of impending failure. There has been a recent uptick in the number of management tools, particularly those based on SaaS offerings, made available by networking hardware vendors that incorporate analytics of this type.
Enhanced Customer Experience
Care agents may offer a better consumer experience by using ML on previous customer care process data at the subscriber level. Similarly, ML can be used to aggregate problems in trouble ticket data based on classification, corrective steps taken, and the efficacy of that step. This closed-loop feedback will assist in enhancing problem-resolution techniques and timelines. Automating service initiation and maintenance operations with sophisticated digital assistants would help field technicians perform their duties more successfully and efficiently.
When It Comes To Networking, What Difficulties Do AI And ML Still Need To Overcome?
Before ML and AI can become commonplace, they must overcome several obstacles. The first is that many businesses still need to embrace automation broadly, which means they may have trouble adopting modern tactics like ML even though those methods offer apparent benefits. Inadequate data collection and partiality in training datasets are additional obstacles that hinder the effectiveness of ML algorithms.
Final Verdict
While data virtualization, configurable networks, and data analytics are used today to automate some tasks, cognitive AI and ML will eventually turn traditional networks into deep learning networks. Historical trends, correlations in large sets of data, and implicit models can all be uncovered with the aid of AI techniques.
As a result of integrating human ingenuity and imagination with the computational power offered by AI, self-improving intelligent algorithms can be created to help in the development of networks shortly. A self-optimizing, self-managing, and self-operating network is possible thanks to the convergence of these methods with the ongoing revolution in networking and connectivity.
AI can reduce trouble tickets and resolve problems even before customers or IT teams recognize them. Root cause analysis and event correlation can use data-mining strategies to identify the network entity related to an issue and remove the network from risk. AI can also reduce human errors by automating network monitoring and management. In the future, AI and ML technology will address many issues in networks and streamline them further for greater efficiency and reduced lag. However, data quality and scalability issues need to be addressed to see further progress in AI and ML.